Unleashing AI for Peace: How Large Language Models Can Mitigate WMD Risks
April 2025
By Yanliang Pan and Daihan Cheng
The popularization of DeepSeek has ushered in a paradigm shift in the field of large language models (LLMs). The prior assumption that only billion-dollar companies with access to troves of computing hardware could train and deploy these powerful frontier artificial intelligence (AI) models simply no longer holds true. Not only is the high-performance Chinese model cheap to train and easy to deploy on local hardware, it is also open source, meaning that anyone can modify its parameters, or fine-tune the model, to perform specific tasks.
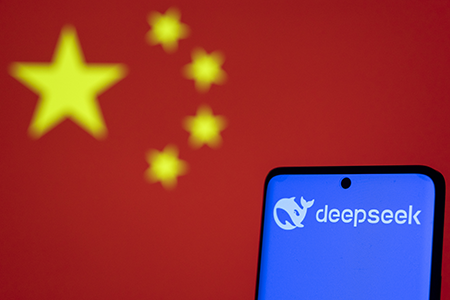
For a nonstate actor seeking to develop a weapon of mass destruction (WMD), such a model would be the perfect assistant. The fact that high-performance frontier AI models no longer have to rely on cloud connection to massive data centers means that the detection of misuse for chemical, biological, radiological, or nuclear (CBRN) proliferation has gone out the window, along with the possibility of enforcing safety guardrails against problematic responses or verifying the model’s end use. Clearly, an approach to AI-CBRN risk governance based on the model of nuclear arms verification or nonproliferation safeguards1 is no longer sufficient. It is time to borrow an alternative frame from humanity’s experience tackling a different existential challenge, namely, that of climate change. That alternative frame is the economics of externalities.
Risks and Governance Challenges
The CBRN risks associated with AI misuse are real and numerous. While investigating its GPT-4 model’s CBRN proliferation potential, U.S.-based OpenAI concluded that “a key risk driver is GPT-4’s ability to generate publicly accessible but difficult-to-find information, shortening the time users spend on research and compiling this information in a way that is understandable to a nonexpert user.”2 Red-teaming exercises also have demonstrated the ability of LLMs to help nonexperts quickly identify dangerous pathogens suitable for a biological weapons attack.3 Similar challenges apply in the chemical and radiological contexts.
Beyond proliferation risks, malicious actors could exploit increasingly capable frontier AI models to augment cyber offensives that compromise the safety and security of critical nuclear infrastructure and supply chains.4 Meanwhile, AI-augmented disinformation and spoofing could increase the risk of inadvertent escalation by distorting states’ perception of their adversaries’ strategic intentions and capabilities. It also could undermine public communication in a CBRN emergency, misleading the response or “worsening the emergency’s consequences” by “[amplifying] public anxiety and [increasing] confusion.”5 The list of CBRN risks associated with AI misuse by malicious actors goes on.
International institutions and multilateral forums specializing in CBRN governance are not ready to confront these emerging risks. Take the nuclear domain as an example. The foundational forums for multilateral deliberations over nuclear disarmament and nonproliferation—including the nuclear Nonproliferation Treaty (NPT) review process, the Conference on Disarmament, and the First Committee of the UN General Assembly—are either trapped in geopolitical gridlock or bound to conservatism by the need for consensus.
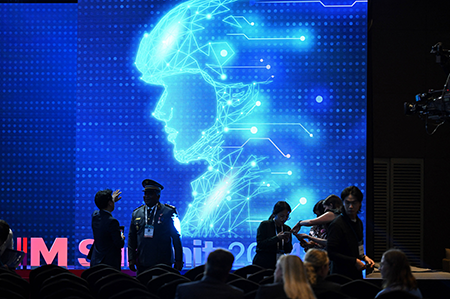
There is also a lack of technical AI expertise among the diplomats attending these forums as AI talent concentrates in the private sector and in national technology labs. Relevant multilateral deliberations, insofar as they have taken place, have focused largely on the risks of military AI, such as AI-automated nuclear-weapon command and control, while failing to address the full range of challenges and opportunities at the AI-nuclear nexus.
Even if diplomats can agree on a broader governance solution, the technology is ultimately developed and deployed by industry and governed by national regulatory authorities. With the former engaged in a race to enhance model performance at lower costs and the latter reluctant to stifle innovation in a national strategic sector, neither has the bandwidth or the incentive to prioritize the mitigation of seemingly remote nuclear risks. Meanwhile, the International Atomic Energy Agency (IAEA), which is traditionally responsible for nuclear safety, security, and safeguards, has neither the capacity to update its safety and security guidance at the pace frontier AI technologies are advancing, nor the mandate to monitor AI models to which nonstate actors may have access, even if such models may serve as proliferation tools.
Balancing AI Externalities With an Economics Frame
An alternative governance framework is needed—one that can regulate the growing AI industry’s international security impact as a global externality. In economics, an externality is a cost or benefit to a third party that is paid for by neither the producer nor the consumer. The classic example of a negative externality is unregulated industrial pollution as it imposes a cost upon the public that is reflected neither in the cost of production nor in the price of the good that the consumer pays. Similarly, unregulated AI advancements could impose public costs in the form of heightened international security risks, requiring government intervention. The trick is to create incentive mechanisms to reward responsible innovation, akin to the incentive schemes that price in the cost of carbon emissions as a global negative externality of polluting industries.
Several such schemes are available. For instance, carbon pricing, implemented through a carbon tax or a cap-and-trade system, assigns a clear monetary price to each unit of carbon emitted, such that heavy emitters pay more to purchase emissions rights whereas those that reduce their carbon footprints receive a credit.6 Carbon offsets, meanwhile, reward carbon reduction activities anywhere—from reforestation to renewable energy investment—by recognizing their positive social impact with a tradable certificate.7 In 2015, economist William Nordhaus introduced club theory to the regulation of carbon emissions, observing that “modest trade penalties on nonparticipants can induce a coalition” more effectively.8
To be sure, these proposals introduce their own implementation challenges, and not all of them are immediately applicable to regulating the AI industry’s CBRN security impacts. Such impacts are still less than fully understood and, in any case, may not be amenable to deterministic quantification. Nevertheless, these challenges do not negate the fundamental rationale for adapting an economics-based regulatory approach to AI-CBRN risks.
Conceptually, these risks are no more and no less than global negative externalities that the industry has imposed upon the public. Although certain AI developers have voluntarily evaluated the international security impact of their frontier models, the practice is neither common nor adequately incentivized. Most importantly, although traditional state-targeted disarmament and nonproliferation approaches such as arms verification and safeguards may prove adequate for CBRN activities already under state government purview, most AI advances are now industry-led rather than state-led, and approaches based on economic incentives are best suited to the private sector.
What would such an approach look like? One option could involve a global tax on AI developers and consumers, designed to address frontier AI’s existential security impact. The amount of such a tax with respect to an AI product would depend on the product’s evaluated potential to contribute to CBRN risks, thus incentivizing the developer to embed safety features or restrict public access to the most dangerous models.
A moderate tax will not hold back industry innovation; indeed, the efficiency gains introduced by DeepSeek’s innovation have cut LLM costs by as much as 96 percent, and further improvements in hardware and algorithm design are expected.9 But such a tax would beneficially shift the responsibility for CBRN risk mitigation to the AI developer as the party most familiar with the model and most capable of implementing access controls. This would reduce the knowledge and expertise asymmetry between regulators and industry. It also would reward responsible approaches to innovation on the part of AI developers who attempt proactively to minimize the technology’s negative global security impact.
The tax revenue may go toward covering the additional national and international proliferation monitoring and counterproliferation expenditures necessary to maintain prior levels of public safety and security. Governments also could identify and reward an exclusive club of responsible AI innovators with tax credits and free-trade benefits in semiconductor hardware, imposing moderate export controls on entities, and tariffs on countries that fail to perform their due diligence to mitigate AI-CBRN risks.
At the same time, the incentive scheme should also include ample opportunities for offsets. Offset arrangements could reward AI developers for making either monetary or in-kind contributions to counterproliferation efforts. These contributions may range from financing nonproliferation research and education through social corporate responsibility programs to developing AI tools that help CBRN professionals tackle CBRN risks more effectively.
Well-designed AI applications can contribute significantly to CBRN risk mitigation. In the nuclear domain, for instance, AI could help enhance threat simulation, analysis, detection, and mitigation at civil nuclear facilities.10 As industry combines generative AI and computer vision for physical security applications, including by using LLMs to query surveillance footage11 and using AI-generated images and videos for the training of computer vision models for surveillance, there is potential for a significant upgrade of the physical protection capabilities of nuclear facilities.
In addition, generative AI is also being integrated into cybersecurity defenses.12 The IAEA is hopeful that “advanced machine learning algorithms” could help identify anomalous cyber activities and prompt the necessary response, despite lingering challenges in data availability and transparency regarding how algorithms arrive at particular outputs.13 When it comes to nuclear security, AI is a source of opportunities as much as it is a source of challenges.
Similarly, AI technology can enhance proliferation detection despite its potential contribution to proliferation risks. The U.S. National Nuclear Security Administration (NNSA), for instance, has used AI to monitor the “nuclear testing and proliferation activities” of U.S. adversaries for years and have continued to develop AI applications in this domain,14 including tools capable of discovering covert fissile material production and nuclear testing, identifying illicit procurement, and tracking technical research that may be useful for a weapons program.15 The Pacific Northwest National Laboratory and Sandia National Laboratories are developing a transformer-based model to detect anomalies in the process data from reprocessing facilities in order to alert IAEA safeguards inspectors about possible diversion.16 The IAEA, meanwhile, has recognized the potential for more advanced AI algorithms to enhance the review of safeguards surveillance footage, enabling earlier and more efficient detection of nuclear facility misuse and material diversion.17
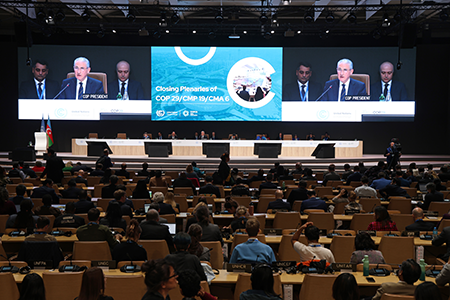
In the export control domain, the latest LLMs, with dramatically improved natural language processing and information retrieval capabilities, could overcome many previously identified limits of export control expert systems designed to advise on compliance.18 AI applications could be used to flag controlled items and investigate end users. They also could review unprecedented volumes of transaction records to identify anomalies, while multimodal LLMs capable of translating visual inputs into natural language could enhance the capacity of border and custom authorities to screen for sensitive items. Companies such as Toshiba already have begun implementing machine learning automated name matching to cross-check end users against export control lists to enhance compliance.19 Machine learning is also helping financial institutions detect illicit money transfers, thus preventing money laundering and terrorist financing, among other activities dangerous to the global community.20
The technologies for building more effective counterproliferation tools are already there. However, the incentive mechanisms that would reward private entities for operationalizing these technological benefits for international security are lacking or absent. In other words, the potential contribution of frontier AI technologies to the global societal good of CBRN security is underpriced. In this context, an offset scheme would reward AI companies for making such contributions while giving them an opportunity to balance out the global CBRN security costs that their innovation incurs, sometimes inevitably.
Multilateral Forums and International Organizations
The legally binding mandate already exists for states to tackle the threat of CBRN proliferation and terrorism by nonstate actors through domestic legislation and multilateral action. UN Security Council Resolution 1540 invokes Chapter VII of the UN Charter to direct that all states “shall adopt and enforce appropriate effective laws” to prohibit CBRN proliferation by nonstate actors “in particular for terrorist purposes, as well as attempts to engage in any of the foregoing activities, participate in them as an accomplice, assist or finance them.”
The same resolution calls upon states to take cooperative action against the threat of WMD proliferation by nonstate actors, including by developing “appropriate ways to work with and inform industry and the public regarding their obligations.” Going forward, state governments should affirm, inside and outside the working process of the 1540 Committee, that the resolution’s mandate extends to the mitigation of AI risks for WMD proliferation by nonstate actors. In addition, they should work toward establishing an incentive-based multilateral framework, supported by national legislation, to price in AI costs and benefits for global CBRN security.
Through further resolutions, the General Assembly could establish a group of governmental experts to develop a clear definition of AI-driven negative and positive externalities for CBRN security and study the feasibility of externality pricing and offset incentives for industry. The group could consider, for instance, ways of evaluating, if not quantifying, various frontier AI models’ contributions to CBRN risks. This exercise could lay the foundation for a global existential security tax or cap-and-trade system while paying particular attention to the impact of controls on developing countries’ equitable access to the peaceful uses of AI technology. The group also could consider implementation issues of a more technical nature, such as compliance monitoring.
International organizations such as the IAEA and the Comprehensive Test Ban Treaty Organization (CTBTO) are already eagerly exploring AI applications for the processing of safeguards and nuclear testing verification data.21 They should continue to leverage established internal mechanisms to operationalize these applications and consider formalizing existing working groups to form AI scientific advisory boards to keep pace with technological advancements. Private sector collaboration is indispensable in this regard. Governments should provide incentives such as tax credits to encourage AI companies to contribute novel solutions and expertise to augment the proliferation monitoring capacity of international organizations as well as the national enforcement of proliferation controls.
Finally, the next NPT Review Conference, in 2026, should consider ways of leveraging beneficial AI applications to further the treaty agenda. It could urge states to report their progress in introducing incentive schemes for regulating the AI industry’s global nuclear security and nonproliferation impact. The conference also could serve as a forum for developed and developing countries to deliberate upon the balance between global controls and equitable access to AI technologies.
In the new era of highly efficient frontier AI models, traditional state-centric CBRN controls are no longer sufficient to mitigate the risks associated with AI misuse by nonstate actors. By treating AI’s potential contribution to CBRN risks as a global negative externality, policymakers can leverage economics-based tools such as taxes, offsets, and club incentives to encourage responsible AI innovation.
Multilateral institutions must also adapt to this new reality by fostering collaboration with the private sector to integrate AI-driven solutions into their activities. Ultimately, the future of CBRN security depends on shaping the direction of AI innovation to balance its positive and negative externalities. A global incentive scheme is among the best ways to mobilize decisive actions before it is too late.
ENDNOTES
1. Sam Altman et al, “Governance of Superintelligence,” OpenAI, May 22, 2023.
2. OpenAI, “GPT-4 Technical Report,” OpenAI, March 2023.
3. Janet Egan and Eric Rosenbach, “Biosecurity in the Age of AI: What’s the Risk?” Belfer Center for Science and International Affairs, November 6, 2023.
4. Vienna Center for Disarmament and Non-Proliferation, “The Impact of Emerging Technologies on the Nuclear Supply Chain,” YouTube, January 17, 2025.
5. Peter Kaiser, “Can You Trust Your Newsfeed? New IAEA CRP Studies How to Mitigate the Harm of Misinformation in Nuclear Emergencies (J15001),” IAEA, January 29, 2019.
6. Jennifer Morris, “Carbon Pricing,” MIT Climate Portal, January 11, 2022.
7. Angelo Gurgel, “Carbon Offsets,” Climate Portal, November 8, 2022.
8. William Nordhaus, “Climate Clubs: Overcoming Free-Riding in International Climate Policy,” American Economic Review 105, No. 4, 2015, pp. 1339-70.
9. Siladitya Ray, “DeepSeek Rattles Tech Stocks: Chinese Startup’s Rise Against OpenAI Challenges U.S. AI Lead,” Forbes, January 27, 2025.
10. National Nuclear Security Administration, Prevent, Counter, and Respond—NNSA’s Plan to Reduce Global Nuclear Threats (Washington, DC: U.S. Department of Energy, 2023).
11. Ashesh Jain, “ChatGPT Meets Video Security: A New Era of Intelligent Surveillance,” Coram, October 30, 2023.
12. Eduard Kovacs, “ChatGPT Integrated into Cybersecurity Products as Industry Tests Its Capabilities,” Security Week, March 9, 2023.
13. Mitchell Hewes, “How Artificial Intelligence Will Change Information and Computer Security in the Nuclear World,” IAEA, June 2023.
14. Rick Perry, “Secretary Perry Addresses the National Security Commission on Artificial Intelligence,” U.S. Department of Energy, November 5, 2019.
15. National Nuclear Security Administration, Prevent, Counter, and Respond—NNSA’s Plan to Reduce Global Nuclear Threats.
16. Steven Ashby, “How PNNL Is Using Machine Learning to Detect Nuclear Threats Quicker and Easier,” Pacific Northwest National Laboratory, March 27, 2023.
17. H. Abdel-Khalik et al., Artificial Intelligence for Accelerating Nuclear Applications, Science and Technology (Vienna: International Atomic Energy Agency, 2022).
18. Rafal Rzepka, Daiki Shirafuji, and Akihiko Obayashi, “Limits and Challenges of Embedding-Based Question Answering in Export Control Expert System,” Procedia Computer Science No. 192, 2021, pp. 2709-19.
19. “Toshiba Strengthens Internal Export Control System with Babel Street Match,” Babel Street, accessed March 1, 2025.
20. Deloitte and United Overseas Bank, “The Case for Artificial Intelligence in Combating Money Laundering and Terrorist Financing,” Deloitte, accessed March 1, 2025.
21. “Science and Technology 2023 Conference: Scientific Advances in CTBT Monitoring and Verification,” CTBTO Preparatory Commission, June 2023.